Serviços Personalizados
Journal
Artigo
Indicadores
-
Citado por SciELO
Links relacionados
-
Similares em SciELO
Compartilhar
Epidemiologia e Serviços de Saúde
versão impressa ISSN 1679-4974versão On-line ISSN 2237-9622
Epidemiol. Serv. Saúde vol.27 no.3 Brasília set. 2018 Epub 10-Ago-2018
http://dx.doi.org/10.5123/s1679-49742018000300010
ORIGINAL ARTICLE
Analysis of the process of malaria transmission elimination with a spatial approach to incidence variation in the Brazilian Amazon, 2016*
1Ministério da Saúde, Secretaria de Vigilância em Saúde, Brasília, DF, Brasil
2Fundação Instituto Oswaldo Cruz, Instituto de Comunicação e Informação Científica e Tecnológica em Saúde, Rio de Janeiro, RJ, Brasil
Objective:
to identify areas where malaria transmission has been eliminated and levels of malaria incidence variation in the Brazilian Amazon in 2016, and to present an indicator of priorities for control actions.
Methods:
an ecological study was conducted with data from the Malaria Epidemiological Surveillance Information System (Sivep-Malaria); municipalities were classified into three groups - elimination achieved, in the process of elimination and in search of reduction -; a composite indicator was created to stratify municipalities prioritized for control actions.
Results:
a total of 337 (41.7%) municipalities were found to have achieved elimination, 398 (49.3%) were in the process of elimination and 73 (9.0%) were in search of reduction; the priority indicator created identified 71 municipalities that accounted for 95% of cases.
Conclusion:
the vast majority of municipalities have already achieved elimination of malaria transmission or are in the process of eliminating transmission; the priority indicator may contribute to targeting malaria control actions.
Keywords: Malaria; Monitoring; Spatial Analysis; Ecological Studies
Introduction
Malaria continues to be a public health problem in Brazil. Responsible for hospitalizations, absence from work and school, as well as deaths, infection affects socioeconomic development in endemic areas. According to the World Health Organization (WHO), in 2015, Brazil reported the highest number of cases of the disease among all the countries of the Americas.1
Malaria incidence is influenced by environmental factors (vegetation, climate and hydrology), sociodemographic factors (migration, population density, level of formal education, culture, income and labor activity of the population), biological factors (species and density of mosquitoes of the Anopheles genus, species of Plasmodium and level of immunity in the population) and political-organizational factors (territorial division, organization and effectiveness of health care services, occupation of the land, agricultural enterprises and infrastructure).2
Efforts to reduce malaria incidence in Brazil date from 1898 through Adolfo Lutz, and 1905 when Carlos Chagas proved household transmission of the disease. In the 1930s the Northeast Malaria Service contributed to eliminating the epidemic in Ceará and Rio Grande do Norte states.3 In the 1940s, 5 million cases of malaria were estimated to have occurred. The Malaria Eradication Campaign began in 1965, and by 1970 its incidence had been reduced to 52,000.4 Since then, there have been successive increases in cases, due to the projects to occupy the brazilian Amazon region that did not take into account the health service structure needed to provide care for people with malaria, culminating with 635,000 cases in the region in 1999.5
Various efforts have been made aimed at controlling Malaria incidence, such as Operation Impact in 1986,6 the Malaria Control Actions Intensification Plan in 1999,7 the new guidelines of the National Program for the Prevention and Control of Malaria (PNCM) in 2003,5 and the partnership with the Global Fund to Fight AIDS, Tuberculosis and Malaria in 2010.8 In 2016, the number of new cases decreased to 143,000. Decentralization of malaria control to states and municipalities with effect from 2000 has also been important for reducing transmission.9 Despite advances, malaria incidence remains high, with more than 99% of the cases concentrated in the brazilian Amazon. The Malaria Eradication Plan (PEM)10 is currently being implemented, containing proposals for the eradication of the disease in the short, medium and long term. However, the use of criteria for prioritizing actions based on a compound indicator, which consider population size, number of cases and incidence of parasitic disease in the municipality, as well as other aspects such as groupings of endemic municipalities and duration of epidemics, could contribute to enhancing PEM.
PNCM has a Malaria Epidemiological Surveillance Information System (Sivep-Malaria)11 as the main tool to support the monitoring and control of the disease. However, statistical tools are required for monitoring of malaria in vulnerable locations, including the identification of the spatial pattern of incidence in these areas. Spatial clustering of disease can be assigned to geaographically overlapping demographic, environmental and/or sociocultural factors, when there is unexpected grouping of cases in space and/or time.12 Thus, knowledge of spatial structure and dynamics is important for the characterization of the region’s health situation,13 better planning and allocation of available resources. Analyses of routine information may assist health service managers in timely identification of epidemics and other indicators, contributing to the effective reduction of disease in municipalities in the brazilian Amazon region.
The objective of this study was to identify areas with eliminated malaria transmission and the levels variation in malaria incidence in the brazilian Amazon in 2016, as well as to present a priority indicator for infection control.
Methods
An ecological study was conducted with data from the Sivep-Malaria system relating to municipalities that make up the brazilian Amazon region.
The region concentrates 99% of malaria cases and covers the states of Acre (AC), Amapá (AP), Amazonas (AM), Mato Grosso (MT), Pará (PA), Rondônia (RO), Roraima (RR), Tocantins (TO) and the western part of Maranhão (MA).14 These nine states have 808 municipalities included in the study area; of these, 52 border with Bolivia, Colombia, Guyana, French Guyana, Peru, Suriname and Venezuela. The Amazon covers 59% of the brazilian territory and, according to the Brazilian Institute of Geography and Statistics (IBGE) population census, it had 25,469,352 inhabitants in 2010.
A quartile control diagram was used to identify variation in malaria incidence levels, containing data on the seven years prior to the 2016 monitoring period, adapted from a study conducted in the municipality of Cruzeiro do Sul (AC).15 The number of autochthonous cases recorded between 2013 and 2016 was ascertained in order to identify municipalities that had achieved transmission elimination and those in the process of eliminating transmission. The annual parasitic index (API), classified as low (0.1 to 9.9 cases per 1,000 inhabitants), medium (10 to 49.9/1,000 inhab.) and high (higher than or equal to 50.0/1,000 inhab.),16 was used to identify the risk of contracting the disease.
An automated algorithm was developed to calculate the lower limits (LLC) and upper limits (ULC) of the control diagram and to determine the number of autochthonous cases, in accordance with meetings held and agreement reached with the PNCM team on 17 January 2017. The results of the algorithm, along with API, enabled the municipalities to be classified into the following groups:
Group 1A - Elimination achieved - municipalities with no records of autochthonous cases during four consecutive years (2013-2016).
Group 2A - In the process of elimination - municipalities with no record of autochthonous cases during three consecutive years (2014-2016).
Group 2B - In the process of elimination - municipalities with no record of autochthonous cases during two consecutive years (2015-2016).
Group 2C - In the process of elimination - municipalities that registered autochthonous cases in the period 2013-2016, but with API≤1 case/1,000 inhabitants in 2016.
Group 3A - In search of reduction - municipalities with API>1 case/1,000 inhabitants, with variation in the number of cases below LLC, and that did not exceed ULC in any week in 2016.
Group 3B - In search of reduction, with stable incidence - municipalities with API>1 case/1,000 inhabitants and with variation in the number of cases between LLC and ULC, during 52 consecutive weeks or more, in 2016.
Group 3C - In search of reduction, with epidemic - municipalities with API>1 case/1,000 inhabitants and with the number of cases above the ULC, in any week in 2016.
The duration of malaria transmission reduction was divided into three categories: (i) short duration = 1-6 weeks of reduction in 2016; (ii) medium duration = 7-24 weeks of reduction; (iii) long duration = 25 or more weeks of reduction.
We used the same definition of an epidemic as Medronho & Perez17 and WHO,18 i.e., 'occurrence of more cases than expected'. All weekly frequency, with one case or more, which exceeded ULC was considered to be an epidemic. The duration of the epidemic was also divided into three categories: (i) short duration = epidemic of 1-6 weeks; (ii) medium duration = 7-24 weeks; (iii) long duration = 25 weeks or more.
The spatial distribution pattern of epidemics in the municipalities was identified using the global and local Moran indexes.19 The spatial dependence of epidemics, statistically represented by spatial autocorrelation, was analyzed by testing the ‘proportion of epidemiological weeks' variable in different locations of space (municipal polygons), comparing the value found in each municipality with the value of the same variable in neighboring municipalities, in the years 2015 and 2016, by means of the global and local Moran indexes.
The Moran Map enabled the visualization of homogeneous areas by distributing the municipalities of the region into quadrants - Q1 +/+, Q2 -/- Q3+/- and Q4 -/+ - as per the method used by Braz et al.2 The global Moran index estimated the extent to which the value of the 'proportion of epidemiological weeks' variable in one municipality was dependent on the values of the same variable in the group of municipalities in the region. Positive values, between 0 and +1, indicated direct autocorrelation; and negative values, between 0 and -1, indicated inverse autocorrelation. The local Moran index identified clusters of epidemic municipalities. The null hypothesis tested was of spatial independence in each municipality, with a significance level of 5%. Cases of malaria notified in 2015-2016 were used to verify which municipalities had persistent direct positive autocorrelation.
We built what was called the 'priority indicator', comprised of six other indicators: (i) number of new cases of malaria in the year; (ii) API; (iii) population size; (iv) proportion of Plasmodium falciparum malaria cases in relation to the total number of cases of the disease; (v) proportion of epidemiological weeks; and (vi) the occurrence of epidemics. This priority indicator was used to classify the municipalities into six hierarchical strata considered to be priorities:
Stratum 1 - municipalities considered to have a high number of cases (>332 cases per year, as per 3rd quartile separator) and also with high API (≥50 cases per 1,000 inhabitants).
Stratum 2 - municipalities considered to have a high of cases and with a large population (≥100,000 inhabitants).
Stratum 3 - municipalities considered to have a high number of cases and with a Plasmodium falciparum proportion ≥ 10%.
Stratum 4 - municipalities considered to have a high number of cases and with direct positive autocorrelation of the proportion of epidemiological weeks (Moran Map quadrant = 1).
Stratum 5 - municipalities considered to have a high number of cases and with recorded epidemics.
Stratum 6 - other municipalities considered to have a high number of cases.
The electronic files containing data on malaria cases were obtained from the Sivep-malaria system on 31 January 2017, with formal PNCM/Ministry of Health authorization. All the analysis of this study considered autochthonous cases of malaria according to the probable municipality of infection.
The population sizes of the municipalities were taken from the Brazilian Unified Health System IT Department (Datasus) website.20 The map layer files were obtained from the Geoprocessing Laboratory of the Institute of Scientific and Technological Health Communication and Information (ICICT), of the Oswaldo Cruz Foundation (Fiocruz).
Data treatment and analysis was performed using Epi Info 3.5.4 (https://wwwn.cdc.gov/epiinfo/html/prevVersion.htm) and TerraView 4.2.2 (http://www.dpi.inpe.br/terraview/php/dow.php?body=DowFiles).
The study was approved by Fiocruz General Postgraduate Coordination/Vice-Chair of Education, Information and Communication on 17/12/2014: Registration No 82957-812014.
As it only used secondary aggregated data, the research project met the ethical considerations provided for by National Health Council (CNS) Resolution No. 466 of 12 December 2012, thus being exempt from assessment by an Ethics Research Committee.
Results
Distribution of the 808 municipalities in the brazilian Amazon according to the level of malaria incidence variation in 2016 identified: 337 (41.7%) municipalities in Group 1A; 54 in Group 2A; 58 in Group 2B; 286 (35.4%) in Group 2C; 15 in Group 3A; none in Group 3B; and 58 in Group 3C. The majority of the Group 1 A municipalities were located in Tocantins (38.0% of the total group), Maranhão (32.3%) and Mato Grosso (24.6%). The Group 1 A municipalities accounted for 50.2% of the total number of municipalities in Maranhão, 58.9% in Mato Grosso and 92.1% in Tocantins. On the contrary, the majority of municipalities with epidemics (Group 3C) were distributed between Amazonas (37.9%), Roraima (15.5%), Amapá (13.8%), Pará (13.8%), and Acre (10.3% of the total group). Stratification of Group 3C identified epidemics in municipalities in Roraima (60.0% of the total number of municipalities), Amapá (50.0%), Amazonas (35.5%), Acre (27.3%), Rondônia (7.7%), Pará (5.6%) and Mato Grosso (0.7%) (Figure 1).
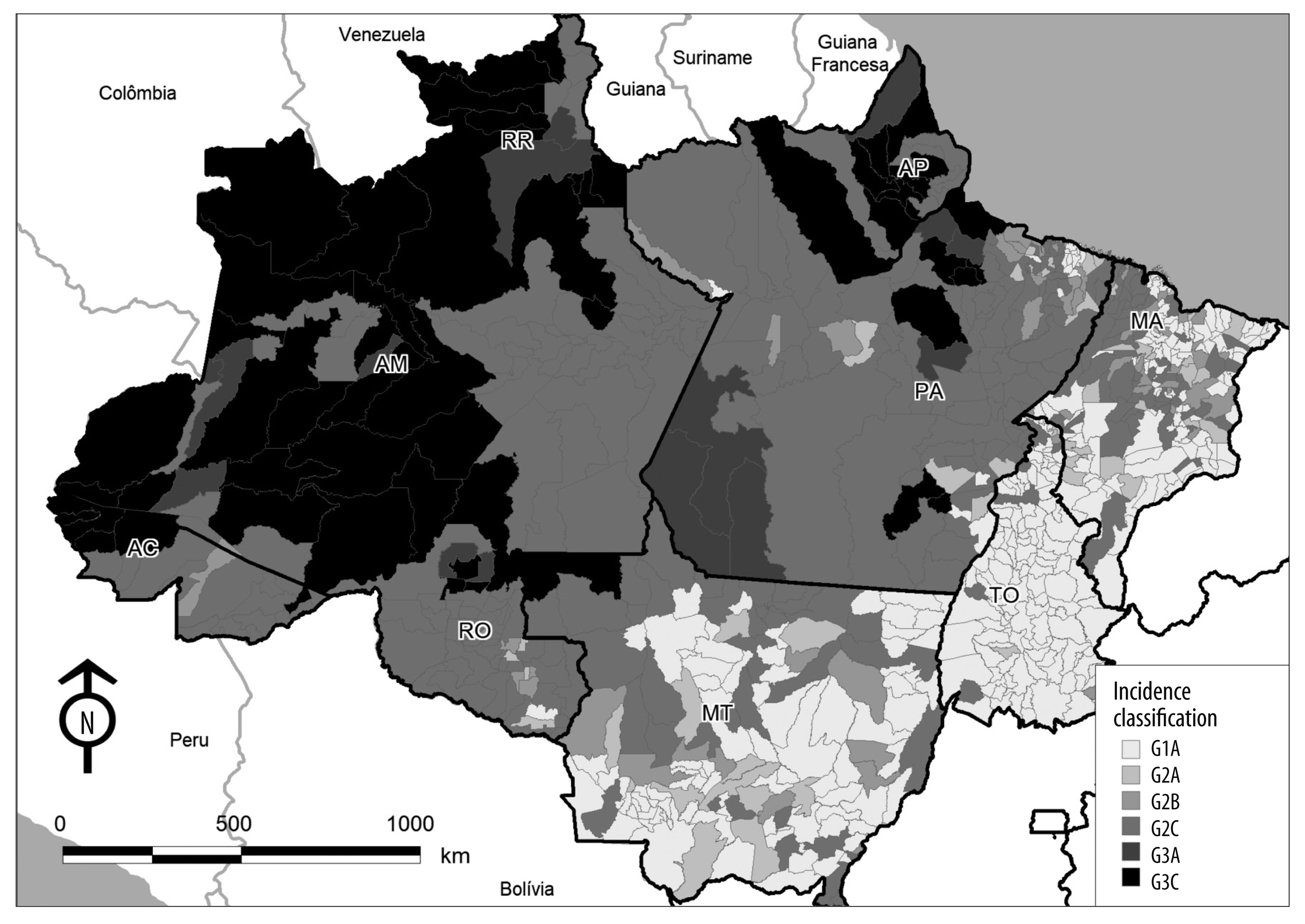
Legend:
G1A = elimination achieved.
G2A = in the process of elimination with no transmission for three years.
G2B = in the process of elimination with no transmission for two years.
G2C = in the process of elimination with IPA≤1 case/1,000 inhabitants.
G3A = in search of reduction, with reduction.
G3C = in search of reduction, with epidemic.
Note: no municipality was classified in group G3B - in search of the reduction; stable incidence.
Figure 1 - Distribution of municipalities according to malaria incidence variation classification, brazilian Amazon, 2016
Analysis of the spatial structure of the malaria epidemics showed that the global Moran index found for the 'proportion of epidemiological weeks' variable was 0.4 in 2015 and 0.5 in 2016 (p=0.01).
In 2015, 57 municipalities were detected as having direct positive autocorrelation (Q1 +/+), 235 municipalities with inverse positive autocorrelation (Q2 -/-) and 15 municipalities with negative autocorrelation (Q4 -/+). There were no municipalities with negative autocorrelation in quadrant 3 (Q3 +/-). 498 municipalities were detected as not having statistically significant autocorrelation (p>0.05). The 57 municipalities in Q1 were located in the states of Acre (n=7), Amazonas (n=29), Amapá (n=8), Pará (n=7) and Roraima (n=6) (Figure 2 - 2015).

Legend:
Q1 (+/+) = direct positive autocorrelation.
Q2 (-/-) = inverse positive autocorrelation.
Q3 (+/-) = negative autocorrelation.
Q4 (-/+) = negative autocorrelation.
Figure 2 - Moran Map for the 'proportion of epidemiological weeks' indicator in endemic area municipalities, brazilian Amazon, 2015-2016
In 2016, the number of municipalities in Q1 was reduced to 46 (19.3%), in relation to the previous year, and a further 225 were classified in Q2. Only two municipalities were found in Q3 and 18 in Q4. The municipalities in Q1 were located in the states of Acre (n=7), Amazonas (n=13), Amapá (n=10), Pará (n=7) and Roraima (n=5) (Figure 2 - 2016). In the same year, 514 municipalities were detected as not having statistically significant autocorrelation (p>0.05).
Direct positive autocorrelation (Q1 +/+) was found to have persisted in 32 municipalities in 2015 and 2016, distributed between Acre (n=5), Amazonas (n=9), Amapá (n=8), Pará (n=7) and Roraima (n=3). In 2015, the number of epidemiologic weeks in these 32 municipalities ranged from 3 in Ferreira Gomes (AP), to 52 in Pedra Branca do Amaparí (AP); the average per municipality was 21 epidemiological weeks with standard deviation (SD) = 14. In 2016 in these 32 municipalities, the number of epidemiological weeks ranged from 3 in Atalaia do Norte (AM) to 51 in São Gabriel da Cachoeira (AM); the average was 20 epidemiological weeks (SD=13).
The 32 epidemic municipalities with persistent direct positive autocorrelation in the two years evaluated formed four distinct clusters. The first cluster (Figure 3 - A1) was comprised of seven municipalities located in Acre (n=5) and Amazonas (n=2), five of which border with Peru: Cruzeiro do Sul, Mâncio Lima and Marechal Thaumaturgo in the state of Acre; and Guajará and Atalaia do Norte in the state of Amazonas. The second cluster (Figure 3 - A2) was comprised of 10 municipalities located in the state of Amazonas (n=7) and Roraima (n=3), two of which border with Colombia - Japurá and São Gabriel da Cachoeira in the state of Amazonas - and three with Venezuela - Barcelos and Santa Isabel do Rio Negro in the state of Amazonas; and Iracema in the state of Roraima. The third cluster (Figure 3 - A3) was comprised of nine municipalities located in the states of Pará (1) and Amapá (8). Of these, the municipality of Almeirim (PA) borders with Suriname, and Laranjal do Jari (AP) borders with French Guiana. The fourth cluster (Figure 3 - A4) was comprised of six municipalities, all in Pará state, none of which is located in the border area.

Legend:
A-1 = Cluster 1.
A-2 = Cluster 2.
A-3 = Cluster 3.
A-4 = Cluster 4.
Figure 3 - Clusters of municipalities with direct positive autocorrelation epidemics - Q1 (+/+) - persistent during two years, brazilian Amazon, 2015-2016
The priority indicator identified 71 municipalities between strata 2 and 6 in 2016. In that year, there were no municipalities in stratum 1. The 71 municipalities registered 136,182 cases of malaria, corresponding to 94.7% of the total for the brazilian Amazon. The population of these municipalities accounted for 19.3% of the region’s total population. Mean API was 25.2 cases per 1,000 inhabitants; and the P. falciparum proportion was 9.9% for the municipalities as a whole (Figure 4 and Table 1).
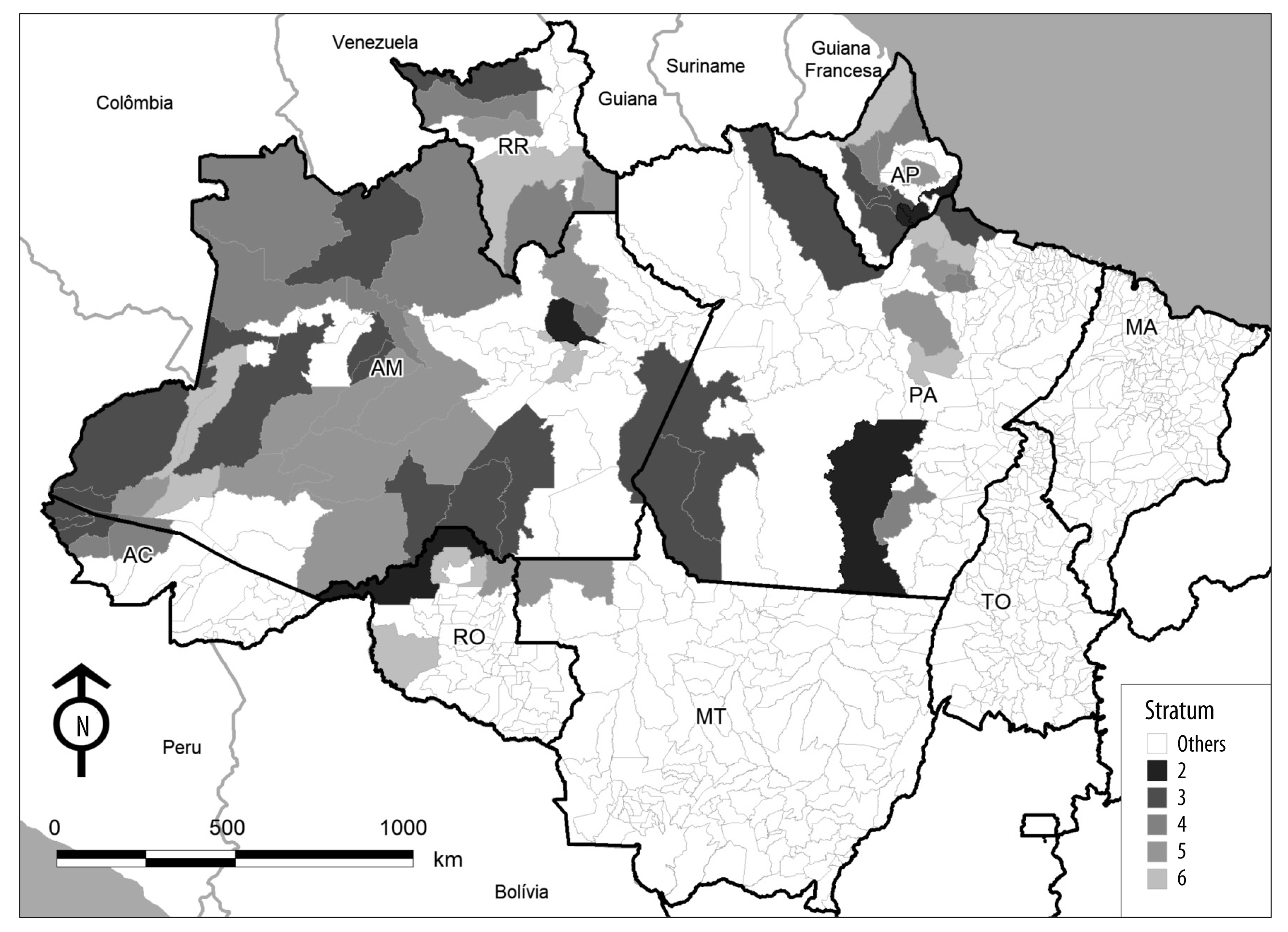
Legend:
Stratum 2 = municipalities with more than 332 cases per year and population ≥100,000 inhabitants.
Stratum 3 = municipalities with more than 332 cases per year and P. falciparum ≥ 10%.
Stratum 4 = municipalities with more than 332 cases per year, and with the quadrant Moran Map = 1.
Stratum 5 = municipalities with more than 332 cases per year and population ≥100,000 inhabitants.
Stratum 6 = other municipalities with more than 332 cases per year.
Others = municipalities with less than 332 cases per year.
Figure 4 - Municipalities classified between strata 2 and 6 (n=71) of the priority indicator and their respective indicators used to calculate malaria control priorities, brazilian Amazon, 2016
Table 1 - Municipalities classified, according to the priority indicator, between 2 and 6 (n=71), and their respective indicators to calculate malaria control priorities, brazilian Amazon, 2016
Code | Municipality | FUa | Population | Populational | Positive slides | IPAb | % of cases per P. falciparum | Number of Epidemiol. Weeks | Moran Map Quadrant | Stratum Priority |
---|---|---|---|---|---|---|---|---|---|---|
130260 | Manaus | AM | 2,094,391 | Large | 10,338 | 0.5 | - | 12 | 1 | 2 |
110020 | Porto Velho | RO | 511,219 | Large | 3,400 | 0.7 | 15.9 | - | 0 | 2 |
160060 | Santana | AP | 113,854 | Large | 1,656 | 1.5 | 1.4 | 14 | 0 | 2 |
160030 | Macapá | AP | 465,495 | Large | 1,297 | 0.3 | 3.1 | 4 | 0 | 2 |
150730 | São Félix do Xingu | PA | 120,580 | Large | 403 | 0.3 | 1.2 | 23 | 1 | 2 |
120020 | Cruzeiro do Sul | AC | 82,075 | Average | 20,969 | 25.5 | 17.7 | 23 | 1 | 3 |
120033 | Mâncio Lima | AC | 17,545 | Small | 8,471 | 48.3 | 13.5 | 43 | 1 | 3 |
120042 | Rodrigues Alves | AC | 17,464 | Small | 6,827 | 39.1 | 22.3 | 47 | 1 | 3 |
130360 | Santa Isabel do Rio Negro | AM | 23,092 | Medium | 2,895 | 12.5 | 23.4 | 43 | 1 | 3 |
130020 | Atalaia do Norte | AM | 18,599 | Small | 2,534 | 13.6 | 19.2 | 3 | 1 | 3 |
160040 | Mazagão | AP | 19,981 | Small | 2,520 | 12.6 | 28.4 | 21 | 1 | 3 |
130165 | Guajará | AM | 16,085 | Small | 2,280 | 14.2 | 19.3 | 21 | 1 | 3 |
150360 | Itaituba | PA | 98,485 | Medium | 2,235 | 2.3 | 20.3 | 0 | 0 | 3 |
160015 | Pedra Branca do Amapari | AP | 14,560 | Small | 2,023 | 13.9 | 10.9 | 29 | 1 | 3 |
130170 | Humaitá | AM | 52,354 | Medium | 1,422 | 2.7 | 22.3 | 19 | 0 | 3 |
140002 | Amajari | RR | 11,285 | Small | 1,128 | 10.0 | 27.2 | 11 | 1 | 3 |
160053 | Porto Grande | AP | 20,143 | Medium | 843 | 4.2 | 20.3 | 2 | 4 | 3 |
150050 | Almeirim | PA | 33,282 | Medium | 807 | 2.4 | 30.0 | 27 | 1 | 3 |
130230 | Jutaí | AM | 16,200 | Small | 730 | 4.5 | 19.0 | 5 | 0 | 3 |
130090 | Canutama | AM | 15,312 | Small | 661 | 4.3 | 31.2 | 13 | 0 | 3 |
130406 | Tabatinga | AM | 62,346 | Medium | 644 | 1.0 | 12.4 | - | 0 | 3 |
130002 | Alvarães | AM | 15,729 | Small | 571 | 3.6 | 16.8 | - | 0 | 3 |
150250 | Chaves | PA | 22,821 | Medium | 531 | 2.3 | 16.4 | 4 | 0 | 3 |
130290 | Maués | AM | 61,110 | Medium | 500 | 0.8 | 21.6 | 9 | 0 | 3 |
130370 | Santo Antônio do Içá | AM | 23,378 | Medium | 494 | 2.1 | 30.8 | 3 | 0 | 3 |
130426 | Uarini | AM | 13,276 | Small | 456 | 3.4 | 16.7 | 4 | 0 | 3 |
150375 | Jacareacanga | PA | 41,487 | Medium | 441 | 1.1 | 10.9 | - | 0 | 3 |
130270 | Manicoré | AM | 53,890 | Medium | 429 | 0.8 | 14.9 | 1 | 0 | 3 |
130380 | São Gabriel da Cachoeira | AM | 43,831 | Medium | 13,130 | 30.0 | 0.1 | 51 | 1 | 4 |
130040 | Barcelos | AM | 27,589 | Medium | 3,946 | 14.3 | 8.2 | 18 | 1 | 4 |
150770 | São Sebastião da Boa Vista | PA | 25,540 | Medium | 2,002 | 7.8 | - | 17 | 1 | 4 |
160020 | Calçoene | AP | 10,345 | Small | 1,816 | 17.6 | 0.9 | 18 | 1 | 4 |
150280 | Curralinho | PA | 32,881 | Medium | 1,371 | 4.2 | 0.3 | 12 | 1 | 4 |
140005 | Alto Alegre | RR | 16,053 | Small | 1,225 | 7.6 | 9.6 | 20 | 1 | 4 |
140047 | Rorainópolis | RR | 27,756 | Medium | 1,139 | 4.1 | 1.2 | 9 | 1 | 4 |
150543 | Ourilândia do Norte | PA | 31,359 | Medium | 1,079 | 3.4 | 0.4 | 45 | 1 | 4 |
160005 | Serra do Navio | AP | 5,025 | Small | 1,040 | 20.7 | 3.6 | 21 | 1 | 4 |
120060 | Tarauacá | AC | 39,427 | Medium | 954 | 2.4 | 7.5 | 4 | 1 | 4 |
130210 | Japurá | AM | 4,660 | Small | 882 | 18.9 | 4.5 | 27 | 1 | 4 |
130356 | Rio Preto da Eva | AM | 31,274 | Medium | 721 | 2.3 | - | 6 | 1 | 4 |
140050 | São João da Baliza | RR | 7,629 | Small | 578 | 7.6 | - | 10 | 1 | 4 |
130280 | Maraã | AM | 18,477 | Small | 490 | 2.7 | 0.4 | 15 | 1 | 4 |
120039 | Porto Walter | AC | 11,059 | Small | 378 | 3.4 | 8.7 | 19 | 1 | 4 |
150180 | Breves | PA | 99,080 | Medium | 2,792 | 2.8 | 0.7 | 23 | 0 | 5 |
130353 | Presidente Figueiredo | AM | 33,703 | Medium | 2,028 | 6.0 | 0.3 | 47 | 0 | 5 |
130240 | Lábrea | AM | 44,071 | Medium | 1,981 | 4.5 | 2.4 | 1 | 0 | 5 |
110013 | Machadinho D'Oeste | RO | 37,899 | Medium | 1,711 | 4.5 | 0.6 | 7 | 0 | 5 |
130120 | Coari | AM | 83,929 | Medium | 1,646 | 2.0 | 0.2 | 2 | 0 | 5 |
130180 | Ipixuna | AM | 27,587 | Medium | 1,565 | 5.7 | 8.2 | 7 | 0 | 5 |
150580 | Portel | PA | 59,322 | Medium | 1,304 | 2.2 | 0.1 | 16 | 0 | 5 |
130420 | Tefé | AM | 62,230 | Medium | 1,114 | 1.8 | 9.2 | 1 | 0 | 5 |
160070 | Tartarugalzinho | AP | 15,665 | Small | 922 | 5.9 | 0.9 | 29 | 0 | 5 |
140030 | Mucajaí | RR | 16,618 | Small | 712 | 4.3 | 4.6 | 20 | 0 | 5 |
510325 | Colniza | MT | 34,885 | Medium | 669 | 1.9 | 0.3 | 2 | 0 | 5 |
130195 | Itamarati | AM | 8,153 | Small | 638 | 7.8 | 5.3 | 3 | 0 | 5 |
130410 | Tapauá | AM | 18,039 | Small | 572 | 3.2 | 1.0 | 1 | 0 | 5 |
130100 | Carauari | AM | 28,111 | Medium | 552 | 2.0 | 1.1 | 5 | 0 | 5 |
140023 | Caroebe | RR | 9,331 | Small | 372 | 4.0 | 0.3 | 33 | 0 | 5 |
150070 | Anajás | PA | 28,012 | Medium | 2,635 | 9.4 | 8.1 | - | 0 | 6 |
110080 | Candeias do Jamari | RO | 24,719 | Medium | 1,253 | 5.1 | 2.2 | - | 0 | 6 |
160050 | Oiapoque | AP | 24,892 | Medium | 922 | 3.7 | 3.4 | - | 4 | 6 |
150030 | Afuá | PA | 37,778 | Medium | 621 | 1.6 | 3.9 | - | 4 | 6 |
150548 | Pacajá | PA | 45,596 | Medium | 614 | 1.3 | 0.7 | - | 0 | 6 |
130140 | Eirunepé | AM | 34,461 | Medium | 605 | 1.8 | 1.7 | - | 0 | 6 |
130390 | São Paulo de Olivença | AM | 37,300 | Medium | 455 | 1.2 | 2.4 | - | 0 | 6 |
130185 | Iranduba | AM | 46,703 | Medium | 414 | 0.9 | 0.0 | - | 0 | 6 |
110094 | Cujubim | RO | 21,720 | Medium | 406 | 1.9 | - | - | 0 | 6 |
140020 | Caracaraí | RR | 20,537 | Medium | 396 | 1.9 | 0.5 | - | 4 | 6 |
110010 | Guajará-Mirim | RO | 47,048 | Medium | 358 | 0.8 | - | 2 | 0 | 6 |
130060 | Benjamin Constant | AM | 40,417 | Medium | 336 | 0.8 | 6.3 | 4 | 0 | 6 |
130110 | Careiro | AM | 36,922 | Medium | 333 | 0.9 | 0.9 | 1 | 0 | 6 |
Total | - | - | 5,413,671 | - | 136,182 | 25.2 | 9.9 | - | - | - |
a) FU: Federative Units.
b) API: annual parasitic index.
Discussion
This study showed that more than 40.0% of the municipalities in the brazilian Amazon have already achieved malaria transmission elimination and these are concentrated in states of Maranhão, Tocantins and Mato Grosso. Almost half the municipalities were in the process of eliminating transmission. Among those which still had transmission, 32 formed persistent epidemic clusters in 2015 and 2016, and require differentiated approaches to infection control. In this sense, the priority indicator grouped the municipalities hierarchically, in order to better direct the control actions and to inform the simultaneous monitoring of malaria incidence variation at municipal, state and national levels, with a view to eliminating disease transmission.
In the municipalities with epidemics, the variability in epidemic duration (between 1 and 51 weeks) may be related to factors such as deforestation of large areas, largescale migration with formation of unprotected settlements, and the lack of health service structure, these being factors identified by two studies: one on the influence of environmental changes on malaria distribution in the brazilian Amazon;21 and the other on the perspective of eliminating malaria transmission in Brazil.22 The 58 epidemic municipalities detected in Group 3C of our study are close to the 50 found by Brazilian Ministry of Health malaria monitoring in the brazilian Amazon in 2015.23
Municipalities with long duration epidemics were more frequent in the states with the highest incidence of malaria, such as Acre, Amazonas, Roraima and Amapá. These epidemics may be related to the operational limitations of health care services, insufficient financial and human resources and, in some localities, inappropriate tools to stop transmission, as noted by the group of experts who presented a draft agenda for the eradication of malaria at the global level.24 Research on malaria incidence trends in the brazilian Amazon between 2004 and 2013, also identified that the four states mentioned in this paragraph are among those where the risk of contracting malaria in the region is greater, despite Roraima having a falling trend in the period.25
The spatial pattern of the distribution of the epidemics in the municipalities formed clusters according to their duration. The global Moran index found that the 'proportion of epidemiological weeks' variable was correlated positively in space, thus confirming the spatial dependence of epidemics among the region’s municipalities. This indicates that malaria transmission in a given municipality, may be related, ecologically, to other neighboring municipalities. This demonstrates the need for integrated malaria control actions between these municipalities. The results found in our study corroborate the findings of a survey on the spatial dependence of malaria epidemics, which indicated autocorrelation in 2003 (0.4), 2007 (0.6) and 2010 (0.5).2
No municipality in the group was detected as having stable incidence, probably due to the cutoff point used for classification (API>1 case/1,000 inhabitants). A study conducted in relation to malaria monitoring in the brazilian Amazon revealed that, generally, municipalities with stable or expected incidence are those with low malaria incidence, this being a situation in which it is possible to plan transmission elimination,26 although requiring increased surveillance and control efforts.
The current situation of malaria surveillance and control requires analysis of various indicators, as shown here. They include indicators already established by PNMC, namely the annual parasitic index (API), the Plasmodium falciparum proportion and the absolute number of cases.23 Spatial autocorrelation of epidemics is another indicator recommended for monitoring the disease.2 Likewise, municipality population size should also be considered, given the possibility of transmission being aggravated in more highly populated areas having favorable environmental conditions for vector proliferation.
The stratified analysis of these indicators offered important inputs for endemic malaria surveillance and control actions. However, examining the indicators together - through the priority indicator - pointed to the relevance of defining priority areas in order to avoid the dispersion of human, financial and logistical resources, since we identified that just 8.3% of the region’s municipalities accounted for 95% of malaria cases. The use of a composite indicator is uncommon in malaria control; however, it has been very important for health surveillance. Examples include its use in the National Immunization Program (Programa Nacional de Imunizações-PNI)27 and in monitoring Brazilian Unified Health System performance.28 Thus, the priority indicator is a possible alternative for informing PNCM and can be adapted if necessary.
This study has some limitations, such as not incorporating cases notified in municipalities located outside of the brazilian Amazon. This information shall be taken into consideration, especially when declaring that municipalities that have achieved malaria transmission elimination. Other important indicators related to vector control were not included because they did not form part of the scope of our analysis.
Following several emergency plans, the incidence of malaria in brazilian Amazon is in the process of effective decline, as indicated by studies on the epidemiological situation of the disease in the region.29 The difficulties facing transmission reduction are greater in places with low transmission, requiring greater surveillance and control organization and effort. This is the great challenge that now imposes itself, with a view achieving United Nations sustainable development goals, especially the goal that foresees the end of epidemics of malaria and other diseases by 2030.29 In this sense, the political-organizational situation may be the differential factor for achieving sustainable malaria transmission elimination in municipalities where this is indicated epidemiologically. Political priority can be sought by state and municipal governments including malaria indicators in annual and pluriannual health plans, ensuring the budgetary and financial resources needed for sustainable malaria monitoring and control actions.
Any lapse in relation to malaria control may cause setbacks in the results achieved, as has already occurred with previous plans to contain the disease. The data for 2017 show a 51% increase in the number of malaria cases reported in the brazilian Amazon in relation to 2016,30) reinforcing the need for new interventions and intensification of malaria surveillance and control actions. Further studies are necessary to verify whether increased infection has occurred in municipalities with persistent transmission or those where malaria transmission had been eliminated.
The current scenario of controlling malaria in brazilian Amazon is promising, but requires the use of new approaches directed to eliminating transmission in the municipalities where it persists. The indicators proposed in this study may broaden the analytical options available for malaria surveillance and control in the brazilian Amazon region.
REFERENCES
1. World Health Organization. World malaria report 2016 [Internet]. Geneva: World Health Organization; 2016 [cited 2018 Apr 6]. 148 p. Available in: Available in: http://www.who.int/malaria/publications/world-malaria-report-2016/report/en/ [ Links ]
2. Braz RM, Guimarães RF, Carvalho Júnior OA, Tauil PL. Spatial dependence of malaria epidemics in municipalities of the Brazilian Amazon. Rev Bras Epidemiol. 2014 jul-set;17(3):615-28. [ Links ]
3. Silveira AC, Rezende DF. Avaliação da estratégia global de controle integrado da malária no Brasil. Brasília: Organização Pan-Americana da Saúde; 2001. [ Links ]
4. Deane LM. Os grandes marcos na história do controle da malária. Rev Soc Bras Med Trop. 1992 out-dez;25(supl 2):12-22. [ Links ]
5. Ministério da Saúde (BR). Secretaria de Vigilância em Saúde. Programa nacional de controle da Malária [Internet]. Brasília: Ministério da Saúde; 2003 [citado 2018 abr 13]. 132 p. Disponível em: Disponível em: http://bvsms.saude.gov.br/bvs/publicacoes/programa_nac_prev_malaria.pdf [ Links ]
6. Loiola CCP, Silva CJM, Tauil PL. Controle da malária no Brasil: 1965 a 2001. Rev Panam Salud Publ. 2002 abr;11(4):235-44. [ Links ]
7. Tauil PL. Avaliação de uma nova estratégia de controle da malária na Amazônia brasileira [tese]. Brasília: Universidade de Brasília; 2002. [ Links ]
8. The Global Fund. Portfolio of grants malaria [Internet]. Geneva: The Global Fund; 2010 [cited 2010 mar 20]. 32 p. Available in: Available in: http://www. theglobal-fund.org/programs/portfolio/?countryID=BRA&lang=en [ Links ]
9. Ladislau JLB, Leal MC, Tauil PL. Avaliação do plano de intensificação das ações de controle da malária na região da Amazônia legal, Brasil, no contexto da descentralização. Epidemiol Serv Saude. 2006 abr-jun;15(2):9-20. [ Links ]
10. Ministério da Saúde (BR). Secretaria de Vigilância em Saúde. Plano de eliminação da Malária no Brasil (Preliminar) [Internet]. Brasília: Ministério da Saúde; 2016 [citado 2017 abr 15]. 38 p. Disponível em: Disponível em: http://portalarquivos.saude.gov.br/images/pdf/2017/janeiro/04/Plano-eliminacao-malaria-pub.pdf [ Links ]
11. Ministério da Saúde (BR). Secretaria de Vigilância em Saúde. Sivep-malária. Sistema de informações de vigilância epidemiológica da malária [Internet]. Brasília: Computorized Systems; 2003 [citado 2018 abr 13]. Disponível em: Disponível em: http://www.saude.gov.br/sivep_malaria [ Links ]
12. Werneck GL, Struchiner CJ. Estudos de agregados de doença no espaço-tempo: conceitos, técnicas e desafios. Cad Saúde Pública. 1997 out-dez;13(4):611-24. [ Links ]
13. Barcellos CC, Sabroza PC, Peiter P, Rojas LI. Organização espacial, saúde e qualidade de vida: análise espacial e uso de indicadores na avaliação de situações de saúde. Inf Epidemiol SUS. 2002 jul-set;11(3):129-38. [ Links ]
14. Brasil. Casa Civil. Lei nº 5.173, de 27 de outubro de 1966. Dispõe sobre o plano de valorização econômica; extingue a Superintendência do Plano de Valorização Econômica da Amazônia (SPVEA); cria a Superintendência de Desenvolvimento da Amazônia (SUDAM), e dá outras providências. Diário Oficial da República Federativa do Brasil, Brasília, DF, 1966 out 31; Seção 1. [ Links ]
15. Braz RM, Duarte EC, Tauil PL. Epidemiology of malaria in the municipality of Cruzeiro do Sul, State of Acre, Brazil, in 2010: uses of a control chart at the local level. Rev Soc Bras Med Trop. 2012 jul-ago;45(4):526-9. [ Links ]
16. Rede Interagencial de Informações para Saúde. Indicadores de dados básicos: conceitos e aplicações [Internet]. Brasília: Rede Interagencial de Informações para Saúde; 2008 [citado 2018 abr 6]. 350 p. Disponível em: Disponível em: http://www.ripsa.org.br/2014/10/30/indicadores-basicos-para-a-saude-no-brasil-conceitos-e-aplicacoes-livro-2a-edicao-2008-2/ [ Links ]
17. Medronho RA, Perez MA. Distribuição das doenças no espaço e no tempo. In: Medronho RA, Carvalho DM, Bloch KV, Luiz RR, Werneck GL. Epidemiologia. São Paulo: Atheneu; 2003. [ Links ]
18. World Health Organization. Disease outbreaks [Internet]. Geneva; 2017 [cited 2017 Nov 8 ]. Available in: Available in: http://www.who.int/topics/disease_outbreaks/en/ [ Links ]
19. Câmara C, Carvalho MS, Cruz OG, Correa V. Análise espacial de áreas. In: Empresa brasileira de Pesquisa Agropecuária - Embrapa. Análise espacial de dados geográficos. Druck S, Carvalho MS, Câmara G, Monteiro AMV, Orgs. Planaltina (DF): Embrapa; 2004. p. 157-206. [ Links ]
20. Ministério da Saúde (BR). Departamento de Informática do SUS. População residente no Brasil, segundo Município. 1996 a 2010 [Internet]. 2010 [citado 2010 fev 2]. Disponível em: Disponível em: http://tabnet.datasus.gov.br/cgi/tabcgi.exe?ibge/cnv/popbr.def [ Links ]
21. Vasconcelos CH, Novo EMLM, Donalisio MR. Uso do sensoriamento remoto para estudar a influência de alterações ambientais na distribuição da malária na Amazônia brasileira. Cad Saúde Pública. 2006 mar;22(3):517-26. [ Links ]
22. Tauil PL. The prospect of eliminating malaria transmission in some regions of Brazil. Mem Inst Oswaldo Cruz. 2011 Jan-Aug;106(Suppl 1):105-6. [ Links ]
23. Ministério da Saúde (BR). Secretaria de Vigilância em Saúde. Malária: monitoramento dos casos no Brasil em 2014. Bol Epidemiol [Internet]. 2015 [citado 2017 set 10];46(25): 1-5. Disponível em: Disponível em: http://portalarquivos2.saude.gov.br/images/pdf/2015/agosto/18/2015-009---Mal--ria-para-publica----o.pdf [ Links ]
24. Alonso PL, Brown G, Arevalo-Herrera M, Binka F, Chitnis C, Collins F, et al. A research agenda to underpin malaria eradication. PLoS Medicine. 2011 Jan;8(1):1-5. [ Links ]
25. Lima ISF, Lapouble OMM, Duarte EC. Time trends and changes in the distribution of malaria cases in the Brazilian Amazon Region, 2004-2013. Mem Inst Oswaldo Cruz. 2017 Jan;112(1):8-18. [ Links ]
26. Braz RM, Duarte EC, Tauil PL. Algoritmo para monitoramento da incidência da malária na Amazônia brasileira, 2003 a 2010. Rev Panam Salud Publica. 2014 jan;35(3):186-92. [ Links ]
27. Braz RM, Domingues CMAS, Teixeira AMS, Luna EJA. Classification of transmission risk of vaccine-preventable diseases based on vaccination indicators in Brazilian municipalities. Epidemiol Serv Saúde. 2016 Oct-Dec;25(4):745-54. [ Links ]
28. Lapouble OMM, Santelli ACFS, Muniz-Junqueira MI. Situação epidemiológica da malária na região amazônica brasileira, 2003 a 2012. Rev Panam Salud Publica. 2015 jul;38(4):300-6. [ Links ]
29. Organização das Nações Unidas. Centro de Informação das Nações Unidas para o Brasil. Transformando nosso mundo: a agenda 2030 para o desenvolvimento sustentável [Internet]. Brasília: Centro de Informação das Nações Unidas para o Brasil; 2015 [citado 2018 abr 6]. 49 p. Disponível em: Disponível em: https://nacoesunidas.org/wp-content/uploads/2015/10/agenda2030-pt-br.pdf [ Links ]
30. Ministério da Saúde (BR). Secretaria de Vigilância em Saúde. Número de casos de malária notificados e diferença percentual entre 2016 e 2018 [Internet]. Brasília: Ministério da Saúde; 2018 [citado 2018 abr 18]. https://public.tableau.com/profile/mal.ria.brasil#!/vizhome/MiniSivep1518_2018_04_10/casos_notificados_2018_regio_Amaznica [ Links ]
*This article is derived a postdoctoral fellowship research conclusion paper entitled 'Implementation and evaluation of the Malaria Incidence Monitoring System (SIMAM) in the Amazon Region: a look at vulnerable populations', presented by Rui Moreira Braz to the Health Information and Communication Postgraduate Program, of the Oswaldo Cruz Foundation 'Brazil without Misery' Postdoctoral Course, in 2017.
Received: January 10, 2018; Accepted: April 10, 2018