Serviços Personalizados
Journal
Artigo
Indicadores
-
Citado por SciELO
Links relacionados
-
Similares em SciELO
Compartilhar
Epidemiologia e Serviços de Saúde
versão impressa ISSN 1679-4974versão On-line ISSN 2237-9622
Epidemiol. Serv. Saúde vol.30 no.4 Brasília dez. 2021 Epub 29-Out-2021
http://dx.doi.org/10.1590/s1679-49742021000400012
EXPERIENCE REPORT
COVID-PA Bulletin: reports on artificial intelligence-based forecasting in coping with COVID-19 pandemic in the state of Pará, Brazil
1Universidade Federal Rural da Amazônia, Campus Paragominas, Paragominas, Pará, Brasil
2Universidade Federal do Oeste do Pará, Programa de Pós-Graduação em Ciências da Saúde, Santarém, PA, Brasil
3Universidade Federal Rural da Amazônia, Campus Parauapebas, Parauapebas, Pará, Brasil
4Universidade Federal do Pará, Instituto de Ciências Biológicas, Belém, PA, Brasil
5Universidade Federal Rural da Amazônia, Instituto Ciberespacial, Belém, PA, Brasil
6Universidade Federal do Paraná, Departamento de Engenharia e Tecnologia Florestal, Curitiba, PR, Brasil
7Universidade Federal de Viçosa, Departamento de Engenharia Florestal, Viçosa, MG, Brasil
8Universidade Federal Rural da Amazônia, Campus Belém, Belém, PA, Brasil
9Universidade Federal do Oeste do Pará, Instituto de Ciências da Educação, Santarém, PA, Brasil
10Universidade Federal Rural da Amazônia, Instituto Socioambiental e dos Recursos Hídricos, Belém, PA, Brasil
Objective:
To report the university extension research result entitled ‘The COVID-PA Bulletin’, which presented forecasts on the behavior of the pandemic in the state of Pará, Brazil.
Methods:
The artificial intelligence technique also known as 'artificial neural networks' was used to generate 13 bulletins with short-term forecasts based on historical data from the State Department of Public Health information system.
Results:
After eight months of predictions, the technique generated reliable results, with an average accuracy of 97% (observed for147 days) for confirmed cases, 96% (observed for 161 days) for deaths and 86% (observed for 72 days) for Intensive Care Unit bed occupancy.
Conclusion:
These bulletins have become a useful decision-making tool for public managers, assisting in the reallocation of hospital resources and optimization of COVID-19 control strategies in various regions of the state of Pará.
Keywords: COVID-19; Artificial Intelligence; Forecast; Neural Networks; Decision Making
Introduction
Coronavirus 2019 (COVID-19) has generated the greatest health and humanitarian crisis of the 21st century, imposing an unprecedented challenge to public health and the world economy,1 demanding permanent epidemiological surveillance.2-5 High mortality rate due to COVID-19 was reported in the North region of Brazil, especially because of the complexity of the Amazon context.6 In the state of Pará, as of February 21, 2021, there were 355,128 Covid-19 cases and 8,278 deaths.7.8 The state of Pará brings together several conditions capable of potentiating the impact of COVID-19, such as deficient healthcare services and limited testing capacity, in addition to the geographical distance between the capital and the municipalities in the interior region of the State.9
Artificial intelligence techniques and mathematical model can be used for Covid-19 monitoring, such as artificial neural networks (ANN), which have as an advantage a practical approach, mathematically rigorous, computationally efficient and capable of assimilating the distortions and uncertainties arising from the data.10-13 The prediction of the prevalence of infectious diseases through ANN has been widely used in epidemiological investigation, both to assess epidemic peaks and to estimate the magnitude of the risk and extension of diseases.14-16
The study aimed to report the university extension research result entitled THE COVID-PA Bulletin, which presented forecasts on the behavior of the pandemic in the state of Pará.
Methods
Context and data collection
This is an experience report with historical data related to the COVID-19 pandemic, obtained from the Pará State Department of Public Health (SESPA) website between March 18, 2020 and August 31, 2020, a period related to the first wave of the pandemic in the state.7
The state of Pará, located in the North region of Brazil, has a territorial extension of 1,245,870 km² and an estimated population of 8,690,745 inhabitants. It has a population density of 6.07 inhabitants per km² and human development index (HDI) of 0.646.18 In Pará, the monthly household income per capita was R$ 883.00 in 2010, when 68.48% of the population lived in urban areas and 31.52% in rural areas.19 SESPA manages the health actions of the Brazilian National Health System (SUS) in 13 Regional Health Centers, distributed by health regions throughout the state of Pará, aiming at decentralizing and regionalizing healthcare service, with 2,057 public health facilities in 2010.7.18.19
Data collection was performed every week, in this study. The data obtained were separated by daily record, corresponding to each day of the week. For each health region, the data collection totaled 147 days of observation for the variable 'confirmed cases', 161 days for 'deaths' and 72 days for the variable 'number of intensive care unit (ICU) beds occupied'.
Data were classified into quantitative and categorical numerical variables, as follows: day of occurrence (date, categorical variable), cumulative cases (quantitative variable), cumulative deaths (quantitative), daily cases (quantitative), daily deaths (quantitative), city names (categorical variable), microregion names (categorical), State health regions (categorical), ICU bed management region (categorical) , municipal demography (quantitative), available ICU beds (quantitative), ICU bed occupancy (quantitative), available hospital beds (quantitative), hospital bed occupancy (quantitative), available doctors (quantitative), available nurses (quantitative), available physiotherapists (quantitative) and available nursing technicians (quantitative).
Research team from the Federal Rural University of The State of Amazônia (UFRA) began a series of studies. A posteriori, the team worked in collaboration with other researchers from the Federal University of Western Pará (UFOPA), Federal University of Pará (UFPA) and State University of Pará (UEPA). They formed a multidisciplinary and interinstitutional team. The scope of the research was to understand the dynamics of transmission and generate predictions capable of identifying patterns in the observed data. Having achieved this purpose, it would be possible to provide reliable information that could assist public health authorities in making important decisions to mitigate the COVID-19 outbreak in a changing environment, where several factors have a positive or negative impact on local and regional transmission.
Use of ANNs for the generation of the COVID-PA Bulletin
In this study, the ANN was used to forecast the number of deaths and confirmed, cumulative and daily cases, and also the demand for ICU beds in the various health regions of Pará. The results were compiled weekly, in format of a bulletin. The set of published bulletins began with a scientific article that proposed a method for generating the first forecasts, by Castro et al.,20 and later, with an article by Braga et al.,21 in order to improve the methods used in this study. The workflow for generating published bulletins followed the steps below:
Data collection on confirmed cases, deaths, hospital resources, in addition to information about the cities;
Data integration and pre-processing, generating the learn table, used for the implementation of the machine learning technique;
Processing/training and selection of the best neural networks;
Generation of case and death forecasts, with a margin of error of 10%;
Analysis of accuracy, residues and error of case and death forecasts;
Storage of post-processing forecasts;
Processing and selection of the best neural networks trained for ICU bed occupancy;
Generation of forecasts of the demand for ICU bed occupancy, with a margin of error of 10%;
Analysis of accuracy, residues and error of ICU bed occupancy forecasts;
Storage of generated data;
Documental compilation and availability of the next issue of the COVID-PA Bulletin on the website of the project,17 and dissemination on social media.
In the Exact Sciences, the use of ANNs is frequent in phenomena that require a process for identifying patterns. The adjustment of different parameters, based on previously known data, enables the model to identify patterns of problems and present, with satisfactory precision, the actual data - observed later. This stage is known as intelligent training system, which requires extensive computational power for processing hundreds of dynamically created ANNs.
The trained ANNs were multilayer perceptron (MLP) type, with a hidden layer.19 The Fletcher-Gloss method was used to define the interval of the number of neurons in the hidden layer, by means of the following equation
where n is the number of input variables in neural networks, n 1 is the number of neurons in the hidden layer and n 2 is the number of neurons in the output layer.
The activation functions tested were exponential, identity, logistics and hyperbolic tangent. The training algorithm used was Broyden-Fletcher-Goldfarb-Shanno (BFGS).20.21 The initial weights of the ANNs were randomly generated. The validation of the ANNs was performed by random partitioning of the data into two sets: training set (80%) and validation set (20%).
After selecting the best trained ANN, the generation and visualization stage of the forecasts started, and then accuracy and error analyses in order to attest the validity of the model, using the following equation,
where R is the real value observed, P the predicted values and N the number of observations. In this article, the term "accuracy" is understood as how close the predilect value is to the actual observed value in percentage values.
Hospital resource forecasts were also estimated in this study. The date of occurrence and the daily deaths forecasted by the best ANN were used as predictor variables to estimate the demand for ICU beds. With the information on the number of ICU beds, it is possible to measure the amount of hospital resources22 and the number health professionals. Thus, a specific ANN was trained to forecast the number of ICU beds needed for the various regions of Pará, and its results were compared with the actual data on ICU bed occupancy. Finally, this information was stored and used to create the charts and tables needed to compile the COVID-PA bulletins.
Publication of COVID-PA bulletins
At first, the bulletins17 were published every seven days, related to the period between March 18, 2020, the date of the first COVID-19 confirmed case in Pará, and July 30, 2020. Subsequently, they were published every 14 days, related to the period from July 31, 2020 to August 31, 2020, the date when the last bulletin was published.
It is worth mentioning that from the third bulletin (05/28/2020), the multidisciplinary team began to present specific forecasts for each of the 13 health regions of Pará. From the sixth bulletin (19/06/2020), forecasts of ICU bed occupancy were also generated, due to the need to monitor this variable, which, at the beginning of the pandemic, was not available periodically. Finally, from the eighth bulletin (06/07/2020), the forecasts for the 40 most populous municipalities or those closer to the State's regional health headquarters were published.
Consequently, after the publication and forwarding of the bulletins to the SESPA, there was a requirement for operational response of distribution of hospital resources and information to support restriction measures and flexibilization of the population mobility. The findings showing that measures were taken after the publication of the bulletins were related to the information about new beds in regions with high pressure on hospitals, directly indicated in the forecasts of this study, and the fact that the study was mentioned by the government dissemination media agency (news about the project: https://pastebin.com/embed_iframe/0mqMEQFY).
This research used only public domain data. There was neither personal information nor human involvement, so it was not necessary to get the approval of a Research Ethics Committee.
Results
The COVID-19-PA Bulletins that were published presented forecasts with an average accuracy of 97% for confirmed cases and 96% for deaths, when compared with the data subsequently observed (Table 1). The forecast of demand for ICU beds was close to the actual number of ICU beds occupied, with an average accuracy equal to 86%. 20-day-or longer forecasts, generated at the beginning of the pandemic, were published in the format of a scientific article.20 Their average accuracy was 96% and 87% for cumulative confirmed cases and cumulative deaths, respectively.
It can be seen that the forecast behavior followed the number of cumulative confirmed cases (Figure 1a). An occurrence was observed in cumulative death forecasts in the second and third bulletins (Figure 1b): between May 19 and 30, 2020, the second bulletin showed an exponential curve, and the third a linear behavior.
The comparative chart of predictions of daily deaths made by ANN for the state of Pará, published in the last bulletin, and its respective observed occurrence, indicate the peak in the number of deaths in the first wave, which coincides, in the month of May, with the period between 5 and 20 (Figure 2).
Figure 3 shows a constant decrease in bed occupancy (in absolute numbers), on an average linear decrease of approximately 3.5 beds per day. Moreover, the forecasts of demands for ICU beds, made by the ANNs, followed this fall.
Table 1 - Accuracies obtained in the scientific article and in the COVID-PA bulletins related to the use of artificial neural networks to forecast confirmed cases, deaths and ICUa bed occupancy, Pará, April 30-August 29, 2020
Technical-scientific article | Publication date | Confirmed cases | Deaths | ICU bed ocuppancya | |||
---|---|---|---|---|---|---|---|
(%) | (%) | ||||||
Scientific Article20 | 04/30/2020 | 96 | 87 | - | |||
Bulletin 1b | 05/13/2020 | 96 | 94 | - | |||
Bulletin 2b | 05/20/2020 | 96 | 93 | - | |||
Bulletin 3b | 05/28/2020 | 94 | 88 | - | |||
Bulletin 4b | 06/05/2020 | 98 | 96 | - | |||
Bulletin 5b | 06/10/2020 | 98 | 97 | - | |||
Bulletin 6b | 06/18/2020 | 98 | 94 | - | |||
Bulletin 7 | 06/26/2020 | 97 | 98 | 87 | |||
Bulletin 8 | 07/06/2020 | 99 | 99 | 85 | |||
Bulletin 9 | 07/14/2020 | 99 | 98 | 91 | |||
Bulletin 10 | 07/21/2020 | 98 | 98 | 93 | |||
Bulletin 11c | 07/31/2020 | 98 | 99 | 90 | |||
Bulletin 12c | 08/14/2020 | 96 | 99 | 62 | |||
Bulletin 13c,d | 08/29/2020 | - | 99 | 93 | |||
General average | - | 97 | 96 | 86 |
a) ICU: Intensive care unit; b) The forecasts of ICU bed occupancy were not presented because initially, this variable was not in the scope of this study; c) The forecasts were made for 14 days, instead of 7 days; d) The forecast of confirmed cases was not generated, due to the change in the testing method for confirmed cases in the state of Pará.
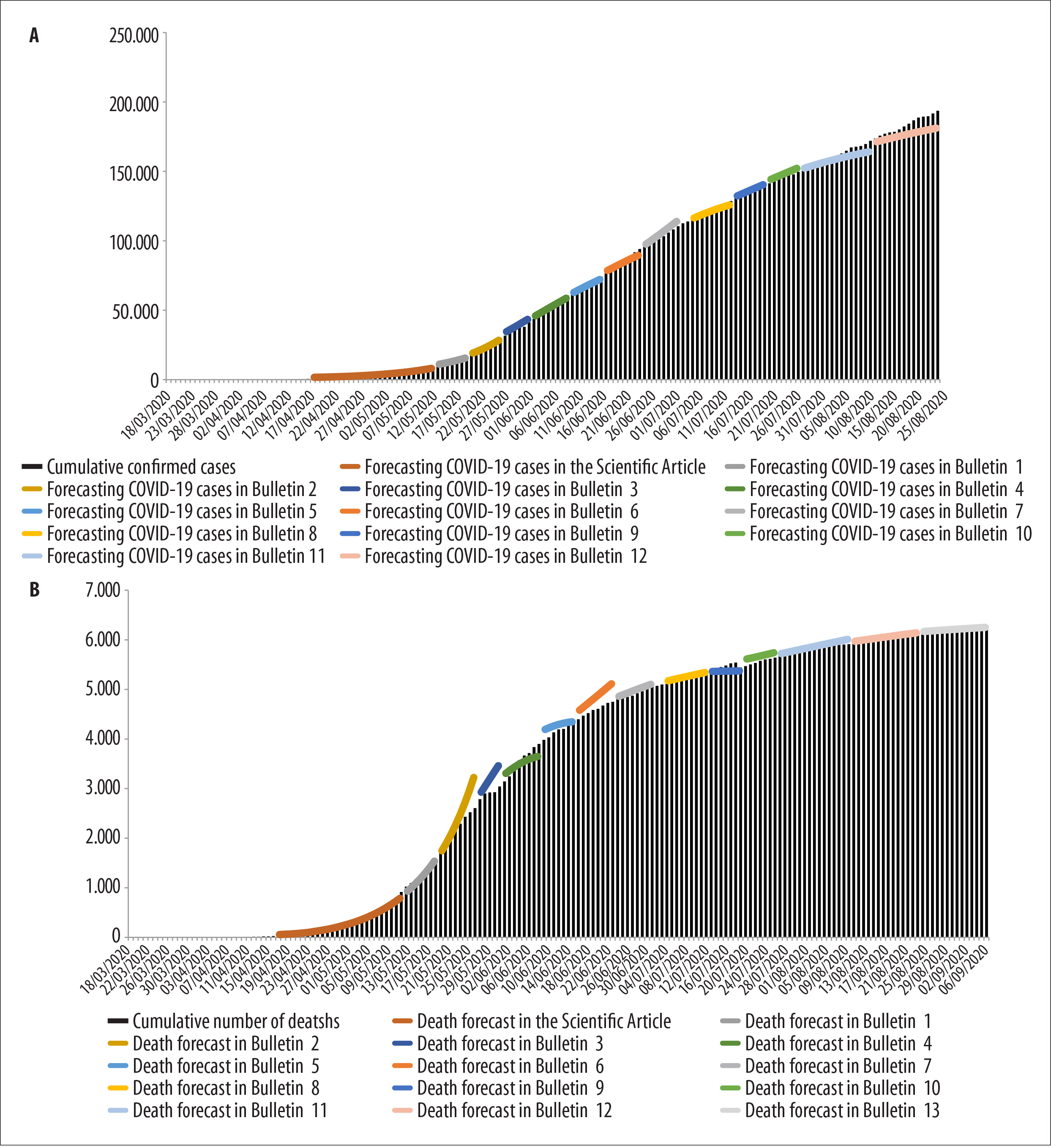
Description: A: Cumulative confirmed cases; B: Cumulative deaths.
Figure 1 - Forecasts generated by artificial neural networks for each COVID-PA Bulletin published in the state of Pará, March 18-September 6, 2020
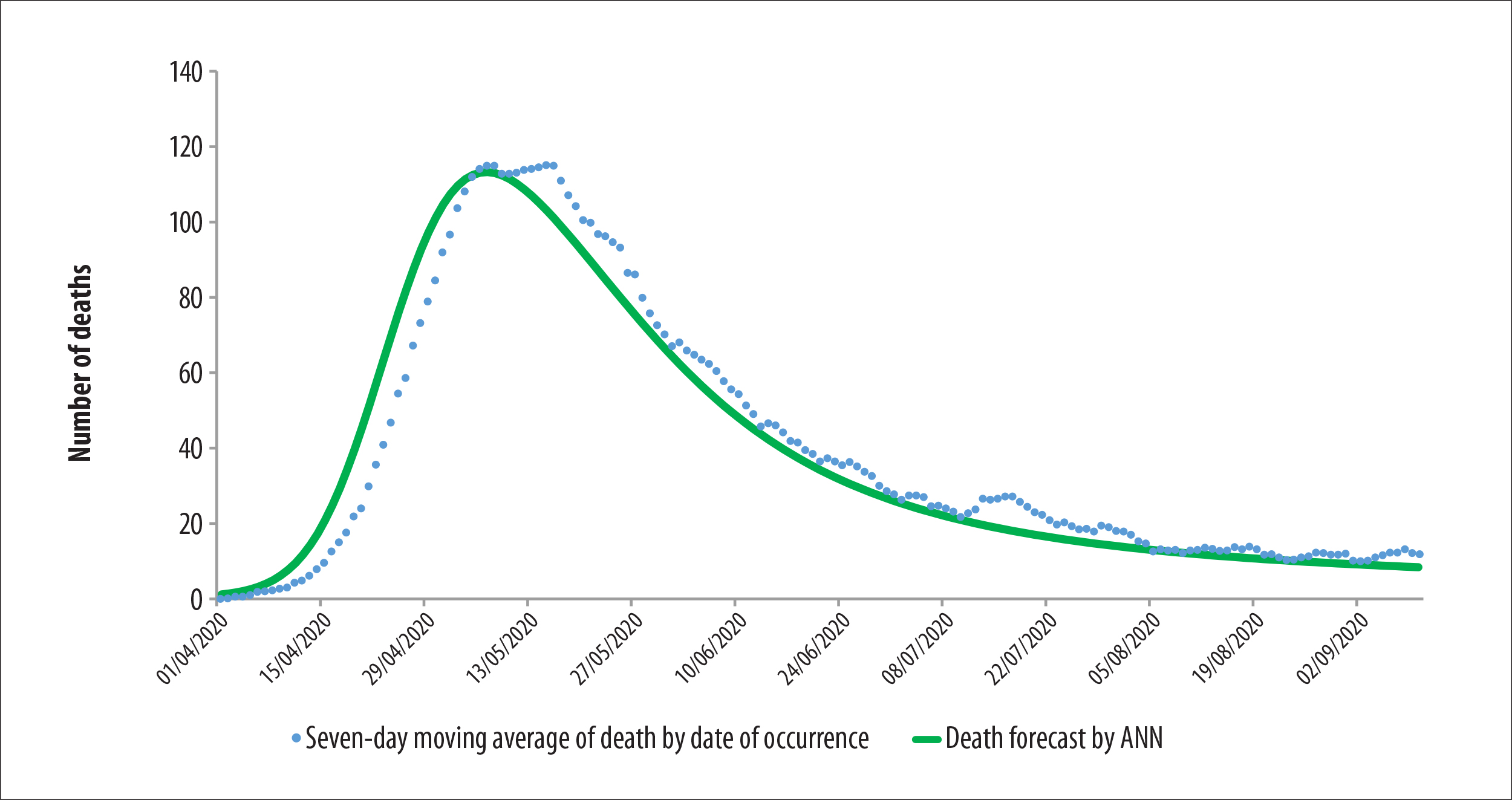
Figure 2 - Moving average of daily deaths and forecast of deaths by artificial neural networks, on data from the COVID-PA bulletins, Pará, April 1-September 2, 2020
Discussion
This article presents an artificial intelligence approach applied to the Covid-19 pandemic data in the state of Pará, resulting in 13 technical bulletins that presented reliable short-term forecasts, with 97% accuracy for confirmed cases, 96% for deaths and 86% for ICU bed occupancy. In the first bulletins, the work team focused on generating forecasts for Pará. When further data on the virus were provided, there was a need to monitor each region, because it could be seen that there were different epidemiological scenarios in the State.
The third and fourth bulletins, published at the end of May and early June, respectively, indicated an incipient decrease in the curve of deaths and daily cases. Several factors may have contributed to this fact, such as government policies (e.g., implementation of mobile clinics with distribution of masks and complementary medicines), adoption of hygiene measures and restriction of the flows of people.23 Population behavior change related to the protection against the virus and the knowledge the health teams acquired regarding the management of COVID-19 cases observed at the end of April and early May, could also explain this reduction. It is worth mentioning that, while some regions in Pará showed stability of COVID-19 cases (for example, the metropolitan region of Belém, capital city of Pará), others were reaching their epidemic peak (Baixo Amazonas, Tapajós and Araguaia), indicating the need for reallocation of hospital resources from one stable region to another with high demand for healthcare services, throughout 2020.
It could be seen that the penultimate bulletin, COVID-PA no. 12, showed a percentage decrease to 62% accuracy regarding the projection of the demand for ICU beds, probably due to the change in the testing method for COVID-19 - from molecular to serological test - from July 31 to August 17, 2020. This fact may have affected the accuracy of bulletin no. 12. Therefore, the input variable 'confirmed cases' was removed from the ANN model, thus the forecast accuracy increased to 93% according to the last bulletin, COVID-PA no. 13.
The number of ICU beds available remained higher than bed occupancy, and higher than the projection of the demand for beds indicated by the bulletins. Meanwhile, the supply of ICU beds began to decrease from August, probably due to the closure of field hospitals for COVID-19 patients in the public network amid dropping COVID-19 cases and deaths.
Artificial neural network technique stores large amount of data. It is used to model the uncertainties associated with predictions. Several studies have applied the same technique during the pandemic aiming at designing the prevalence and identifying the seasonality of the disease.24-26 Thus, taking into consideration the transmissibility of SARS-CoV-2, it becomes necessary to periodically observe the substantial increase in ICU bed capacity for a rapid patient isolation,27 through initiatives that have already been implemented and reported, based on historical data.28.29 This quantitative structure, in spatial and temporal scale, provides useful information to assess the impact of control interventions, and how behavior changes affect transmission dynamics.13.30
The data used in the training phase resulted in a model dependence on the collected data retrieved from secondary sources. They were comprised of confirmed cases, deaths and ICU beds. This condition implies periodic adjustment of model parameters. However, long-term forecasts, subsequently evaluated, showed that this limitation did not significantly alter the results of this study.
The short-term forecasts using the ANNs, fulfilled the function of predicting the disease outbreak behavior, helping prevent health system collapse in the state of Pará, in 2020, especially in terms of reallocation of hospital beds. Their efficiency helped in public manager decision-making, in addition to keeping people informed about the epidemiological behavior of the disease. Finally, the COVID-PA bulletins, produced by this university extension research, showed that partnerships among universities, health surveillance and state management are fundamental for the production of relevant information, in a critical period of pandemic, contributing to protect people’s lives in the capital and interior region of Pará.
Acknowledgements
The authors wish to thank all health professionals who contributed with information about the care on the front line of the pandemic, especially Andrea A. F. de Souza, Cristina Shizuka K. Castro and Helloyza H. F. A. Pompeu and also Ben R. S. Pereira and Jean Patrick C. Souza, for the technical and administrative support of the Federal Rural University of the Amazônia for the publication of the COVID-PA bulletins.
REFERENCES
1. Li L, Huang T, Wang Y, et al. COVID-19 patients’ clinical characteristics, discharge rate, and fatality rate of meta‐analysis. J Med Virol. 2020;92(6):577-583. doi:10.1002/jmv.25757 [ Links ]
2. Croda J, Oliveira WK de, Frutuoso RL, et al. Covid-19 in Brazil: Advantages of a socialized unified health system and preparation to contain cases. Rev Soc Bras Med Trop. 2020;53. doi:10.1590/0037-8682-0167-2020 [ Links ]
3. Takemoto MLS, Menezes M de O, Andreucci CB, et al. The tragedy of COVID-19 in Brazil: 124 maternal deaths and counting. Int J Gynecol Obstet. Published online July 29, 2020:ijgo.13300. doi:10.1002/ijgo.13300 [ Links ]
4. Islam N, Shabnam S, Erzurumluoglu AM. Temperature, Humidity, and Wind Speed Are Associated with Lower Covid-19 Incidence. Cold Spring Harbor Laboratory Press; 2020. doi:10.1101/2020.03.27.20045658 [ Links ]
5. Lima NT, Buss PM, Paes-Sousa R. A pandemia de COVID-19: uma crise sanitária e humanitária. doi:10.1590/0102-311X00177020 [ Links ]
6. Cavalcante JR, Cardoso-Dos-Santos AC, Bremm JM, et al. COVID-19 no Brasil: evolução da epidemia até a semana epidemiológica 20 de 2020. Epidemiol e Serv saude Rev do Sist Unico Saude do Bras. 2020;29(4):e2020376. doi:10.5123/s1679-49742020000400010 [ Links ]
7. Pará Information and Communication Technology Company. Monitoramento COVID-19 (SESPA). Empresa de Tecnologia da Informação e Comunicação do Pará - PRODEPA. Published 2020. https://www.covid-19.pa.gov.br/ [ Links ]
8. Pará’s Government. Decreto Estadual No609 de 16 de abril de 2020. LEGIS-PA. Published 2020. Accessed August 5, 2020. https://www.sistemas.pa.gov.br/sisleis/legislacao/5444 [ Links ]
9. Universidade Federal de Pelotas. COVID-19 No Brasil: Várias Epidemias Num Só País: Primeira Fase Do EPICOVID19 Reforça Preocupação Com a Região Norte.; 2020. Accessed July 22, 2020. https://wp.ufpel.edu.br/covid19/files/2020/05/EPICOVID19BR-release-fase-1-Portugues.pdf [ Links ]
10. Luengo-Oroz M, Hoffmann Pham K, Bullock J, et al. Artificial intelligence cooperation to support the global response to COVID-19. Nat Mach Intell. 2020;2(6):295-297. doi:10.1038/s42256-020-0184-3 [ Links ]
11. Massad E, Burattini MN, Lopez LF, Coutinho FAB. Forecasting versus projection models in epidemiology: The case of the SARS epidemics. Med Hypotheses. 2005;65(1):17-22. doi:10.1016/j.mehy.2004.09.029 [ Links ]
12. Al-qaness MAA, Ewees AA, Fan H, Abd El Aziz M. Optimization Method for Forecasting Confirmed Cases of COVID-19 in China. J Clin Med. 2020;9(3):674. doi:10.3390/jcm9030674 [ Links ]
13. Mohamadou Y, Halidou A, Kapen PT. A review of mathematical modeling, artificial intelligence and datasets used in the study, prediction and management of COVID-19. Appl Intell. 2020;50(11):3913-3925. doi:10.1007/s10489-020-01770-9 [ Links ]
14. Manliura Datilo P, Ismail Z, Dare J. A Review of Epidemic Forecasting Using Artificial Neural Networks. Int J Epidemiol Res. Published online 2019. doi:10.15171/ijer.2019.24 [ Links ]
15. Wahyunggoro O, Permanasari AE, Chamsudin A. Utilization of Neural Network for Disease Forecasting. In: Proceedings 59th ISI World Statistics Congress. ; 2013:549. Accessed July 12, 2020. https://www.semanticscholar.org/paper/Utilization-of-Neural-Network-for-Disease-Wahyunggoro-Permanasari/88b515658b38e404dfffe8fdc55da519076c848d [ Links ]
16. Fernandes R. Compartmental Epidemiological Models for Covid-19: Estimation, Goodness-of-Fit and Forecasting Epidemics | IEEE Latin America Transactions. Special Issue on Fighting against COVID-19. Published 2020. Accessed October 5, 2020. https://latamt.ieeer9.org/index.php/transactions/article/view/4399 [ Links ]
17. Universidade Federal Rural da Amazônia. Boletim COVID-19 PARÁ. PROEX - Pro-reitoria de Extensão. Published 2020. Accessed October 20, 2020. https://proex.ufra.edu.br/index.php?option=com_content&view=article&id=265:boletim-covid-ufra&catid=2&Itemid=375 [ Links ]
18. Instituto Brasileiro de Geografia e Estatistica - IBGE. IBGE | Cidades@ | Pará | Panorama. Published 2020. Accessed June 3, 2021. https://cidades.ibge.gov.br/brasil/pa/panorama [ Links ]
19. Governo do Estado do Pará. Plano Estadual de Saúde.; 2016. Accessed June 3, 2021. https://www.conass.org.br/pdf/planos-estaduais-de-saude/PA_Plano-estadual-saude-2016-2019.pdf [ Links ]
20. Castro J, Souza GN, Brito SR, et al. Redes neurais artificiais na previsão de contágio e óbitos por COVID-19: um estudo no Estado do Pará, Brasil. Int J Dev Res. 2020;10(4):35416-35421. Accessed October 20, 2020. https://www.journalijdr.com/redes-neurais-artificiais-na-previsão-de-contágio-e-óbitos-por-covid-19-um-estudo-noestado-do-pará [ Links ]
21. Braga MB, Fernandes RS, Souza GN, et al. Artificial neural networks for short-term forecasting of cases, deaths, and hospital beds occupancy in the COVID-19 pandemic at the Brazilian Amazon. Zeng Q, ed. PLoS One. 2021;16(3):e0248161. doi:10.1371/journal.pone.0248161 [ Links ]
22. Moghadas SM, Shoukat A, Fitzpatrick MC, et al. Projecting hospital utilization during the COVID-19 outbreaks in the United States. Proc Natl Acad Sci U S A. 2020;117(16):9122-9126. doi:10.1073/pnas.2004064117 [ Links ]
23. Mecenas P, Bastos R, Vallinoto A, Normando D. Effects of Temperature and Humidity on the Spread of COVID-19: A Systematic Review. Cold Spring Harbor Laboratory Press; 2020. doi:10.1101/2020.04.14.20064923 [ Links ]
24. Yang Z, Zeng Z, Wang K, et al. Modified SEIR and AI prediction of the epidemics trend of COVID-19 in China under public health interventions. J Thorac Dis. 2020;12(3):165-174. doi:10.21037/jtd.2020.02.64 [ Links ]
25. Saba AI, Elsheikh AH. Forecasting the prevalence of COVID-19 outbreak in Egypt using nonlinear autoregressive artificial neural networks. Process Saf Environ Prot. 2020;141:1-8. doi:10.1016/j.psep.2020.05.029 [ Links ]
26. Tamang SK, Singh PD, Datta B. Forecasting of Covid-19 cases based on prediction using artificial neural network curve fitting technique. Glob J Environ Sci Manag. 2020;6(Special Issue (Covid-19)):53-64. doi:10.22034/GJESM.2019.06.SI.06 [ Links ]
27. Grasselli G, Pesenti A, Cecconi M. Critical Care Utilization for the COVID-19 Outbreak in Lombardy, Italy. JAMA. 2020;323(16):1545. doi:10.1001/jama.2020.4031 [ Links ]
28. Li R, Rivers C, Tan Q, Murray MB, Toner E, Lipsitch M. The demand for inpatient and ICU beds for COVID-19 in the US: lessons from Chinese cities. medRxiv. Published online March 16, 2020:2020.03.09.20033241. doi:10.1101/2020.03.09.20033241 [ Links ]
29. Jiang X, Coffee M, Bari A, et al. Towards an Artificial Intelligence Framework for Data-Driven Prediction of Coronavirus Clinical Severity. C C. 2020;63(1):537-551. doi:10.32604/cmc.2020.010691 [ Links ]
30. Chowell G, Sattenspiel L, Bansal S, Viboud C. Mathematical models to characterize early epidemic growth: A review. Phys Life Rev. 2016;18:66-97. doi:10.1016/j.plrev.2016.07.005 [ Links ]
Received: February 26, 2021; Accepted: July 02, 2021